All explanatory variables used in our empirical model are not strictly exogenous. If this is the case, straightaway panel estimation will yield biased results. Therefore, a test for endogeneity should be applied. If the null hypothesis of exogeneity is rejected, then Arellano– Bover (1995)–Blundell Bond (1998) system GMM estimator will be employed. GMM allows for controlling the joint endogeneity of explanatory variables through the use of internal instruments (see Enders, 2004 and Baltagi, 2005). The tricky issue in GMM methodology is to select valid instruments/moments. No rule of thumb exists in instruments' selection. For this purpose, Murray (2006) discussed various tricks. Two diagnostic tests are there for the validity of instruments. Firstly, we have Hansen test for over-identifying restrictions with the null hypothesis that the instruments are not correlated with the residuals. Secondly, we have Arellano–Bond test for second-order correlation in the first differenced residuals. The advantage of using GMM over the other instrumental variable (IV) methods is that a G MM estimator is more efficient if heteroskedasticity is present. Similarly, a GMM estimator is not worse than an IV estimator if heteroskedasticity is not present. In this study, the lagged values of independent variables have been used as instruments. We also perform a robustness check by the inclusion of additional control variables in our model.
We apply the mentioned estimation technique in this study on aggregated and disaggregated data (by decomposing global sample into various groups like Asia, Europe, and Africa) for various periods (1996-2017, 2013-2017, and 2008-2012). The intention for this disaggregation is to watch the role of corruption in growth and public debt relationship for BRI countries closely. Similarly, the purpose of dividing period is to see the impact of BRI initiative on the relationship.
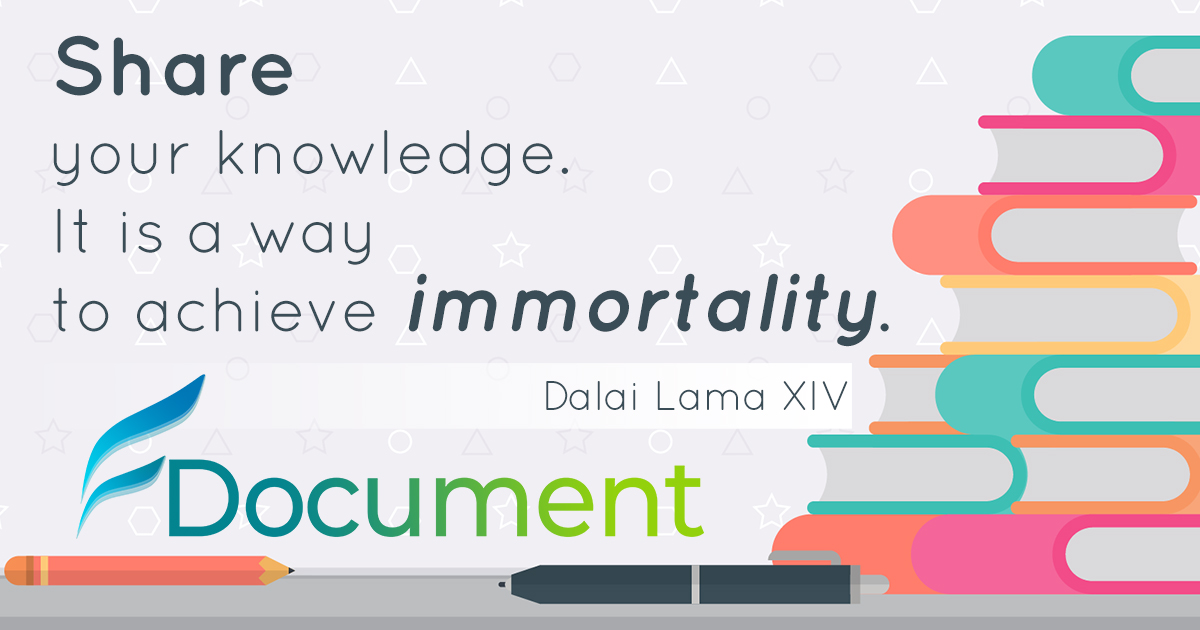
To see the impact of BRI on growth, we use fixed and random effect models by including
period dummy on the aggregate data (global sample). For this purpose, we will
divide data into two parts, i.e., 2008-2012 and 2013-2017, by assigning 0 to
the former and 1 for the later. Comparative to the random and fixed effect
models that are not restricted, the pooled model is restricted and assumes that
countries are homogeneous. When it is necessary to control for omitted
variables that are constant over time but differ between countries, the
fixed-effects model is desirable. Since the fixed effect considers
heterogeneity and individual country effects, therefore, it gives better
estimates than the pooled model.
On the other hand, no individual country effects
are assumed in the random effect model. Hausman (1978) test is employed to
choose between fixed- and random-effect models. Hausman test specifies whether
the explanatory variables are correlated with specific effects or not. Hausman
test makes sure the selection of the model with consistent results. Random
effects are not correlated with the explanatory variables is the central
assumption in random effect estimation. The fixed effect model is feasible if
the p-value is significant, i.e., < 5%. On the other hand, if it is greater
than 5%, then the most appropriate model is the random-effects model.
Furthermore, we employ quantile regression (QR) to examine influence of public debt on
income distribution in BRI countries. For comparison purpose, we also employ
the fixed effect model. QR estimation methodology enables the estimation at
different intervals of condition distribution.
No comments:
Post a Comment